
In the opinion of the Null hypothesis, is this test statistic "extreme"?ģ) Calculate the p-value: what's the probability of getting a test statistic as extreme or MORE extreme than the one you got?Ĥ) If the p-value is less than or equal to the significance level - reject the null hypothesis. 5% is a popular choice, as is 1% and even 10%, if you can live with yourself knowing you are wrong 10% of the time.Ģ) Calculate your test statistic. So we can compute, for example, that the probability that xbar is within 1 SE of mu is roughly 68%, within two SE's is roughly 95% and within 3 is roughly 99.7%.ġ) Choose a significance level - the probability that we will reject the null hypothesis, even though its true (oops!). (The SD of a estimator, like Xbar, is called the Standard Error, or SE). We know, however, that Xbar is NOT equal to mu, it is merely an estimate that is unbiased (so it likes to "hang out" around mu) and has a relatively small standard error (so it doesn't stray too far from mu - and in fact, the larger the sample size, the closer Xbar tends to stay to mu.) But we can be even more precise because we assumed the population was normal, Xbar is also normal with mean mu and SD equal to sigma/sqrt(n). Our alternative is called the "Alternative Hypothesis." Because the null hypothesis represents conventional wisdom, it gets the benefit of the doubt, and will be overturned only with exceptional evidence to the contrary.īecause we want to know about the mean of the population, we estimate using one of our favorite estimators, the average Xbar (sum of the observations divided by the number of observations). But that's a fine point for later.) The "conventional wisdom" is called the Null Hypothesis. This gives us an advantage over the conventional wisdom that some would say is unfair in some contexts. (Note that our options are much more vague than the conventional wisdom. 2) mu is greater than mu0 3) mu is less than mu0. (For example, conventional wisdom claims that the mean is 0.) We believe instead that something else is true.

The conventional wisdom says that the mu is a particular value, let's call it mu0. We are interested in the value of the mean of the population, mu. We've collected data on a single variable which we assume has a normal distribution with mean mu and SD sigma. So we need to understand how our sample will vary. Because the sample is random (and because the population has a variety of values), we can't count on getting the exact same values if we repeat our experiment. And we test our hypothesis on this random sample. To test our hypotheses about the value of these parameters, we take a random sample of independent observations. For example, if I'm running for president, I want to know that my support is NOT less than 50%.
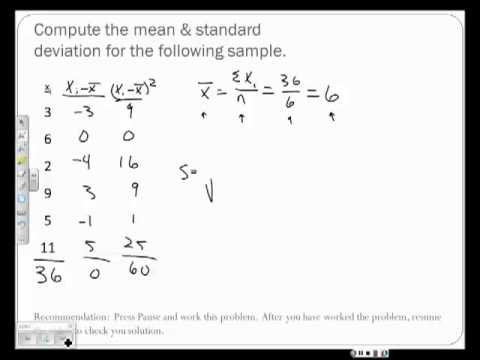
In hypothesis testing, we are actually more interested in what value the parameters aren't. The probability distribution of this population can be summarized with one (or maybe two or three) parameters, and we wish to know what these parameters are. # "as the size inceases further, we would expect sigma-hat to settle down # at a value close to the overall sigma-hat" (Wetherill and Brown, 1991, # p.General Framework: There exists a population of wildly exciting observations, but, alas, the population is too large to observe them all.
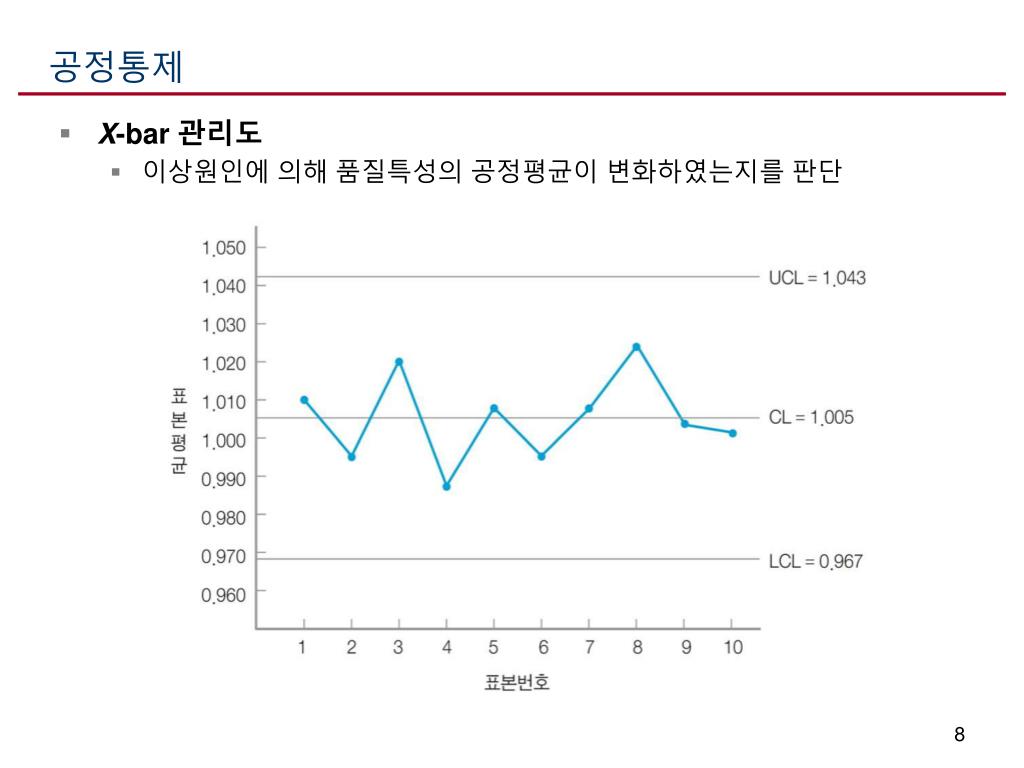
# 2) using SD qcc(x, type= "xbar.one", std.dev = "SD", data.name= "Water content (in ppm) of batches of antifreeze" ) # the Shewhart control chart for one-at-time data # 1) using MR (default) qcc(x, type= "xbar.one", data.name= "Water content (in ppm) of batches of antifreeze" ) # Water content of antifreeze data (Wetherill and Brown, 1991, p.
